Multiple regression is an excellent tool to predict variance in an interval dependent variable based on linear combinations of the interval dichotomous or dummy independent variables. Updated on September 12 2018.
Sem With Amos From Zero To Hero 3 Cb Sem Vs Pls Sem Youtube
How significantly different are the observed covariance structure and the covariance structure implied by the anatomical model and 2 the difference between.
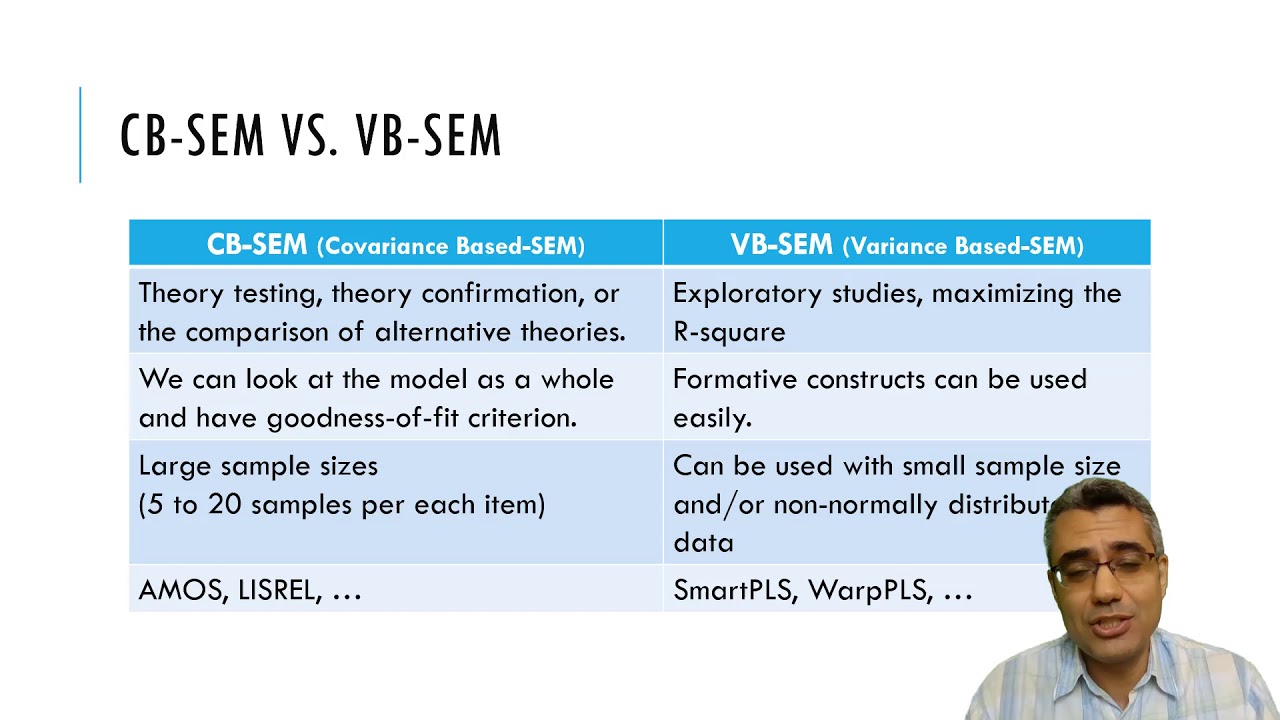
. It is interpreted as the proportion of total variance that is explained by the model. Typically these relationships cant be statistically. Goodness-of-fit Criteria Typically in SEM statistical inference is used to measure.
Use of SEM for mediation analysis. We then later use these two matrixes and the differences between them to calculate indexes of model fit. Basically I want to see whether adding this extra predictor allows us.
5 variances 10 covariances and 5 means. The difference between PCA and EFA can be quite hard to intuitively grasp as their output is very familiar. Structural Equation modeling however relies on several statistical tests to determine the adequacy of model fit to the data.
In statistics confirmatory factor analysis CFA is a special form of factor analysis most commonly used in social research. You can compute the number of parameters in a saturated model of k observed variables by the formula k k12 k. Acceptable fit indices do not imply the relationships are strong.
This is sometimes done if it is believed that the two variables have something in common that is not captured by the latent variables. How SEM Works You supply two main things Formal specification of model Observed relationship between variables ie a covariance or correlation matrix You also need to supply the number of participants or cases Model implies a set of covariances Software tries to reproduce observed covariance matrix. If the model is acceptable researchers then establish whether specific paths are significant.
It is a test of the null hypothesis that the model-implied covariance matrix hatboldsymbolSigmaboldsymboltheta equals the population covariance. 12 Model chi-square goodness of fit. Indeed high fit indices are often easier to obtain when the relationships between.
Go to my three PowerPoints on Measuring Model Fit in SEM small charge. I am doing some structural equation modeling. Y distribution is assumed to be standardized.
The log likelihood for this model is -29432087. I have made one model with 3 exogenous variables predicting 5 endogenous variables and would like to compare that to a second model that is identical except it contains one more exogenous variable a fourth. This means that computation of the percentage variance explained by a certain factor is simply using the squared loading times the factor variance.
The most essential component of a structural equation model is covariance or the statistical relationship between items. Fit refers to the ability of a model to reproduce the data ie usually the variance-covariance matrix. Structural Equation Modeling SEMis quantitative research technique that can also incorporates qualitative methods.
Figure 1 shows a path diagram for the causal relationships between the three variables in the smoking prevention example discussed earlier. In our example the expression y1 y5 allows the residual variances of the two observed variables to be correlated. In structural equation modeling the fit indices establish whether overall the model is acceptable.
The proper selection of methodology is a crucial part of the research study. In our example it is 5 512 5 20. Most of the models that you will see in the literature are SEM rather than path analyses.
The idea is that PCA aims at extracting the most variance possible from all variables of the dataset whereas EFA aims at creating consistent factors from the dataset without desperately trying to represent all the variables. Structural Equation Modeling SEM is a second generation multivariate method that was used to assess the reliability and validity of the model measures. Adjusted R-squared should always be used with models with more than one predictor variable.
For the saturated model we estimated 20 parameters. Structural equation model fit. It is used to test whether measures of a construct are consistent with a researchers understanding of the nature of that construct or factor.
Likewise it will increase as predictors are added if the increase in model fit is worthwhile. Statistically appraising the fit of a model to the covariance matrix is accomplished using a goodness of fit test referenced against the χ2 distribution which takes as its argument. You can calculate the degrees of freedom for your SEM by subtracting the number of parameters you wish to estimate--the unknowns--from your total pieces of knowns.
Y variance which is 1 if no covariates gives the proportion variance. When modelling data using structural equation modelling SEM one or more models may be fit to the same covariance matrix. 1 the goodness of the overall fit of the model ie.
The true population covariance denoted Sigma is called the variance-covariance matrixSince we do not know Sigma we can estimate it with our sample and call it hatSigmaS or the sample variance-covariance matrixThe function cov. The main difference between the two types of models is that path analysis. If your unknowns exceed your knowns your model will be.
The chi-square test indicates the amount of difference between expected and observed covariance matrices. Each statistical technique has certain characteristics that determine applicability to a given problem. A good-fitting model is one that is reasonably consistent with the data and so does not necessarily require respecification.
Both can be called variants on the probit model but theta parameterization corresponds more exactly to the probit regression estimates in which the. Jan 31 2013. Structural Equation Modeling SEM Path analysis is a special case of SEM.
The model chi2 test is the most common global fit index in SEM and is a component of several other fit indices. Prevention program x i social norm z i and amount of smoking y iIn this example all variables that are effected by other variables social norms and amount of smoking are endogenous. There are situations in which a high R-squared is not necessary or relevant.
Dividing by the item variance ie. SEM is used to show the causal relationships between variables. As such the objective of confirmatory factor analysis is to test whether the data fit a hypothesized.
Variance to 10 estimating the measurement residual variance. The relationships shown in SEM represent the hypotheses of the researchers. The lavaan package automatically makes the distinction between variances and residual variances.
To test how well our model compares to a. A chi-square value close to zero indicates little difference between the expected and observed covariance matrices. The variance-based SEM is working with component weights that maximize variance whereas the covariance-based SEM is based on factors that.
These scaling choices are arbitrary in the sense that the chi-. Path analysis contains only observed variables and has a more restrictive set of assumptions than SEM.
Structural Equation Modeling Sem
How To Chose Sem Amos Or Sem Smartpls For Data Analysis
Under What Conditions Should A Researcher Use Variance Based Sem Vs Covariance Based Sem
Model Fit Indices And Recommended Values For Sem Analysis Kline 2005 Download Table
0 Comments